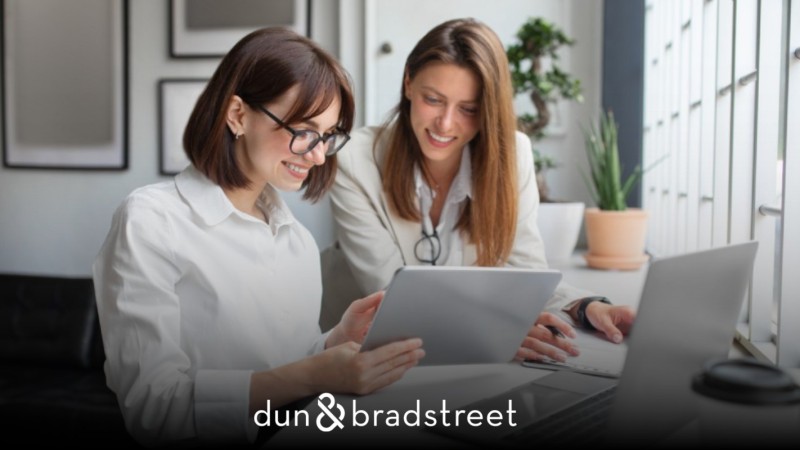
Master Data Management is a comprehensive approach to managing an organization’s critical data assets. It involves the processes, governance, policies, and technologies that ensure the consistency, accuracy, and accountability of data across an organization. MDM focuses on creating a single, authoritative source of master data—data that is critical to the operations and reporting of a business, such as customer information, product details, supplier records, and financial data.
The primary goal of MDM is to eliminate data silos and inconsistencies, providing a unified view of critical data that can be trusted and leveraged across various business functions. This unified view is essential for effective analytics, as it allows organizations to make decisions based on accurate and complete information.
The Importance of Data Quality in Analytics
Analytics is fundamentally dependent on data quality. Poor quality data can lead to inaccurate analyses, misguided decisions, and ultimately, business failures. Data quality dimensions include accuracy, completeness, consistency, timeliness, and relevance. MDM plays a vital role in enhancing these dimensions by establishing robust data governance and management processes.
- Accuracy: MDM ensures that master data is accurate by implementing validation rules and data cleansing processes. By regularly monitoring and updating master data, organizations can significantly reduce errors and inconsistencies. Accurate data is essential for generating reliable analytics, as any inaccuracies can lead to faulty conclusions.
- Consistency: MDM creates a single source of truth for master data, ensuring that all departments access the same information. This consistency is critical for analytics, as it enables decision-makers to rely on uniform data across different systems and reports. When various departments work with inconsistent data, it can lead to conflicting analyses and hinder collaboration.
- Completeness: MDM promotes data completeness by identifying missing or incomplete data fields and implementing processes to fill these gaps. Complete datasets enable more comprehensive analyses, allowing organizations to uncover insights that might otherwise be overlooked.
- Timeliness: Timely data is essential for effective decision-making. MDM processes ensure that master data is regularly updated, reflecting the most current information available. This is particularly important in fast-paced industries where timely insights can significantly impact competitiveness.
Enhancing Data Accessibility for Analytics
Data accessibility is another critical factor in the relationship between MDM and analytics. MDM systems facilitate the organization, storage, and retrieval of master data, making it easier for analysts and decision-makers to access the information they need.
- Centralized Data Repositories: MDM solutions often create centralized repositories for master data, enabling users to access a comprehensive view of critical information without having to sift through multiple databases. This centralization not only enhances accessibility but also improves data governance, as organizations can enforce security and access controls more effectively.
- Data Integration: MDM systems facilitate data integration by connecting various data sources and systems. This integration allows organizations to combine data from disparate systems, creating a holistic view that supports more robust analytics. When data from various sources is integrated and harmonized, analysts can generate insights that take into account a wider range of factors.
- Self-Service Analytics: Modern MDM systems often incorporate self-service analytics capabilities, empowering users to explore and analyze master data without relying solely on IT. This democratization of data access allows business users to derive insights more quickly, enhancing agility and responsiveness in decision-making.
Supporting Advanced Analytics and Business Intelligence
As organizations increasingly adopt advanced analytics techniques, including machine learning and predictive modeling, the role of MDM becomes even more critical. High-quality master data serves as the foundation for these advanced analytics initiatives.
- Machine Learning Models: Machine learning algorithms require high-quality input data to generate accurate predictions. MDM ensures that the data fed into these models is reliable and relevant, improving the effectiveness of machine learning initiatives. Inaccurate or inconsistent data can lead to biased models and unreliable outcomes.
- Business Intelligence (BI) Reporting: MDM enhances BI reporting by providing a single source of truth for master data. This consistency enables organizations to generate accurate and insightful reports that drive strategic decision-making. With reliable master data, BI tools can deliver meaningful visualizations and insights that empower executives and managers.
- Customer Insights: For businesses focused on customer analytics, MDM is particularly valuable. By maintaining accurate and consistent customer data, organizations can better understand customer behavior, preferences, and needs. This understanding allows for targeted marketing strategies, improved customer experiences, and ultimately, increased loyalty.
Conclusion
The relationship between Master Data Management and analytics is symbiotic; effective MDM enhances the quality, consistency, and accessibility of data, leading to improved analytical outcomes. In a world where data is abundant but often unreliable, organizations that invest in robust MDM practices will be better positioned to leverage their data assets for strategic advantage. By ensuring that master data is accurate, consistent, and readily accessible, organizations can unlock the full potential of analytics, driving better decision-making and fostering innovation. As the landscape of data continues to evolve, the importance of MDM will only grow, making it an essential component of any successful data strategy.